Jeffrey O. Kephart
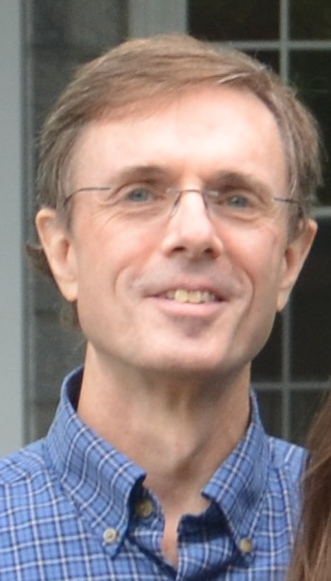
Titel:
Viewing Autonomic Computing through the Lens of Embodied Artificial Intelligence
Abstract:
Embodied AI is the study of systems that interact with people in physical environments to assist them with cognitive tasks, while autonomic computing is concerned with systems that manage their own behavior in accordance with high-level objectives. While they differ in detail, at the right level of abstraction the two fields have much in common. Both seek to extend human cognitive abilities by leveraging an understanding of human objectives, and for both the natural architecture is a self-managing multi-agent system. Accordingly, it is worthwhile to consider how insights gained from each field might be applied to the other.
In this talk, I will focus on two lessons from embodied AI that I believe can be applied fruitfully to autonomic computing. After illustrating a few embodied AI agents in action, I will use those examples as a basis for questioning an assumption for which I have historically been a strong advocate: that administrative objectives can be specified in terms of pre-defined utility functions. A second issue raised by embodied AI is that there is a natural tension between situating compute power at the edge to meet latency requirements and situating it in the cloud for scalability. I will describe some interesting autonomic computing research challenges that arise in this context.
Bio:
is a distinguished research scientist at IBM Research in Yorktown Heights, NY. Known for his work on computer virus epidemiology and immune systems, electronic commerce agents, autonomic (self-managing) computing systems, and data center energy management, he presently leads research in the area of embodied AI systems. His work has been featured in Scientific American, The New York Times, Wired, Forbes, The Atlantic Monthly, Discover Magazine, and comparable publications. He has co-authored over 60 patents and 150 papers, which have received over 20,000 citations. In 2013, he was awarded the rank of IEEE Fellow for his leadership and research in founding autonomic computing as an academic discipline. He graduated from Princeton University with a BS in electrical engineering (engineering physics) and received his PhD from Stanford University in electrical engineering, with a minor in physics. Jeffrey O. Kephart