Prof. Dr. Karl Aberer
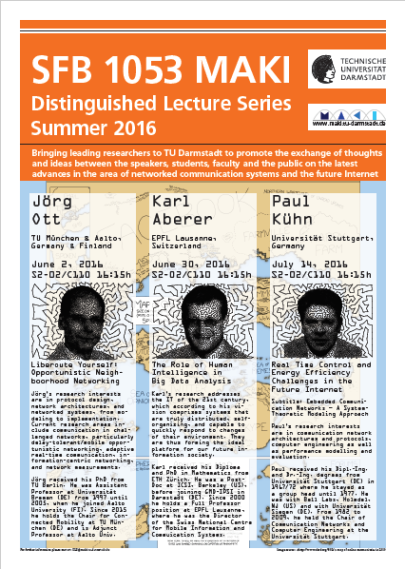
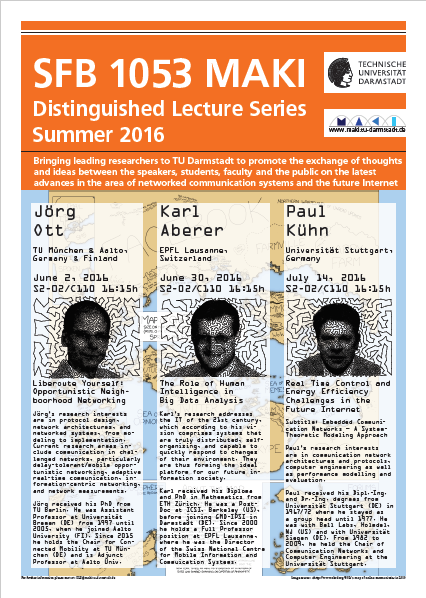
30 June 2016, 04:15 pm
“The Role of Human Intelligence in Big Data Analysis”
Venue: S2|02 room C110 (Robert-Piloty building, Hochschulstr. 10, 64289 Darmstadt)
Abstract:
Big Data has become in the recent years central to our information society. Data is being considered as the key economic resource of information society, often compared with the importance of oil for the industrial society. The collection and analysis of massive datasets has become a central factor and driver for transformation for many sectors of industry. Different technological advances are driving these developments, such as increased connectivity, the ability to handle massive data in modern cluster computing centres and the rapidly growing power of machine intelligence due to improvements in algorithms and the ability to analyse increasingly large datasets.
The key challenge in Big Data Analysis is the creation of useful knowledge from data. For a long term there has been a disconnection between industry and research, on how this challenge is tackled. Whereas research has been focusing on the use of automated methods based on data mining and machine intelligence, industry has been relying in business intelligence largely on rule-based approaches, meaning that rules are engineered by human experts [1]. With the rapidly increasing power of machine intelligence, adopted in particular by the big Web companies, e.g., in advertising, this traditional view starts to shift and even turn into quite extreme viewpoints. The need for understanding causal relationships has been proclaimed by some as obsolete, and to be replaced by the pure use of correlations [2]. In other words, human understanding and reasoning should be replaceable by the use of statistical patterns.
We claim that despite the impressive developments in machine intelligence the use of human intelligence will remain a crucial factor. The key question is not whether the one can replace the other, but how they work together in a productive and efficient way. There are good reasons to believe that human input will remain essential, in particular in areas where domain specific or contextual knowledge is required. In general, machines cannot learn about intentions, valuations and interpretations of humans or human experts, unless massive behavioural traces are collected, which is possible in exceptional cases (e.g. Google search logs that are exploited for advertisement), but not in settings involving deep domain knowledge. This observation is also supported by the rapid adoption of crowd-sourcing platforms, such as CrowdFlower and Amazon Mechanical Turk, enabling the execution of human intelligence tasks at massive scale.
We will illustrate this claim through three exemplary recent research works that illustrate different models of interaction of human and machine intelligence.
In the first case we see how human intelligence is need in order to enable machine intelligence. We show this for the problem of evaluating credibility of Web contents, e.g. providing information on health and nutrition [3]. Evaluating credibility relies both on domain expertise, for evaluating factual correctness of information, and on social factors, for establishing common beliefs of what is considered as accepted truth. Ground truth based on human input is primordial to enable any form of automated credibility evaluation. Based on human annotated document corpuses we identified document features that are strong indicators of credibility and serve as the basis for automated credibility evaluation based on supervised learning and recommender algorithms.
In the second case we provide an example of how the computational power of machines and human intelligence can work interactively together to solve difficult intelligence problems. We consider a task that is of primordial importance in the processing of Big Data, the integration of heterogeneous datasets that come from different sources [4]. Using automated analysis of large amounts of structural and content features of database schemas and content allows nowadays too come up with reasonably good suggestions for correlating heterogeneous data, but the correctness of those suggestions is still largely insufficient for data integration. Thus human expertise and judgement remains an unavoidable component in establishing correct semantic relationships for heterogeneous data. We show how to optimize human interventions, in verifying automatically produced semantic relationships, and thus to significantly speed up the data integration process performed in collaborative manner between human and machine intelligence.
In the third example we show of how human interpretation is needed in order to derive useful insights from automatically analysed Big Data sets for the case of Social Media analysis. Using content and network analysis allows us nowadays to discover a wide range of latent structures in social media data, such as topics, communities and events. Linking such findings to specific business questions remains a challenging task that can only solved by the human experts. We demonstrate from a recent project with a major food company, what are possible findings that can be derived from Social Media and the Web through automated analysis with subsequent human interpretation.
In conclusion, there is a huge potential in optimizing the interaction of human and machine intelligence. Automated processing of large amounts of data is a great tool for discovering hidden structures and correlations that are inaccessible to humans. However, both for setting the right targets for analysis and for selecting and interpreting the results, humans will remain key in many domains, in particular those requiring specialized expertise such as science and business.
[1] Chiticariu, Laura, Yunyao Li, and Frederick R. Reiss. “Rule-Based Information Extraction is Dead! Long Live Rule-Based Information Extraction Systems!.” EMNLP. No. October. 2013.
[2] http://www.wired.com/2008/06/pb-theory/)
[3] Olteanu, Alexandra, et al. “Web credibility: Features exploration and credibility prediction.” Advances in Information Retrieval. Springer Berlin Heidelberg, 2013. 557-568.
[4] Nguyen, Quoc Viet Hung, et al. “Pay-as-you-go reconciliation in schema matching networks.” Data Engineering (ICDE), 2014 IEEE 30th International Conference on. IEEE, 2014.